New research on social media during the 2020 election, and my predictions
This is crossposted from Statistical Modeling, Causal Inference, and Social Science.
Back in 2020, leading academics and researchers at the company now known as Meta put together a large project to study social media and the 2020 US elections — particularly the roles of Instagram and Facebook. As Sinan Aral and I had written about how many paths for understanding effects of social media in elections could require new interventions and/or platform cooperation, this seemed like an important development. Originally the idea was for this work to be published in 2021, but there have been some delays, including simply because some of the data collection was extended as what one might call “election-related events” continued beyond November and into 2021. As of 2pm Eastern today, the news embargo for this work has been lifted on the first group of research papers.
I had heard about this project back a long time ago and, frankly, had largely forgotten about it. But this past Saturday, I was participating in the SSRC Workshop on the Economics of Social Media and one session was dedicated to results-free presentations about this project, including the setup of the institutions involved and the design of the research. The organizers informally polled us with qualitative questions about some of the results. This intrigued me. I had recently reviewed an unrelated paper that included survey data from experts and laypeople about their expectations about the effects estimated in a field experiment, and I thought this data was helpful for contextualizing what “we” learned from that study.
So I thought it might be useful, at least for myself, to spend some time eliciting my own expectations about the quantities I understood would be reported in these papers. I’ve mainly kept up with the academic and grey literature, I’d previously worked in the industry, and I’d reviewed some of this for my Senate testimony back in 2021. Along the way, I tried to articulate where my expectations and remaining uncertainty were coming from. I composed many of my thoughts on my phone Monday while taking the subway to and from the storage unit I was revisiting and then emptying in Brooklyn. I got a few comments from Solomon Messing and Tom Cunningham, and then uploaded my notes to OSF and posted a cheeky tweet.
Since then, starting yesterday, I’ve spoken with journalists and gotten to view the main text of papers for two of the randomized interventions for which I made predictions. These evaluated effects of (a) switching Facebook and Instagram users to a (reverse) chronological feed, (b) removing “reshares” from Facebook users’ feeds, and (c) downranking content by “like-minded” users, Pages, and Groups.
My guesses
My main expectations for those three interventions could be summed up as follows. These interventions, especially chronological ranking, would each reduce engagement with Facebook or Instagram. This makes sense if you think the status quo is somewhat-well optimized for showing engaging and relevant content. So some of the rest of the effects — on, e.g., polarization, news knowledge, and voter turnout — could be partially inferred from that decrease in use. This would point to reductions in news knowledge, issue polarization (or coherence/consistency), and small decreases in turnout, especially for chronological ranking. This is because people get some hard news and political commentary they wouldn’t have otherwise from social media. These reduced-engagement-driven effects should be weakest for the “soft” intervention of downranking some sources, since content predicted to be particularly relevant will still make it into users’ feeds.
Besides just reducing Facebook use (and everything that goes with that), I also expected swapping out feed ranking for reverse chron would expose users to more content from non-friends via, e.g., Groups, including large increases in untrustworthy content that would normally rank poorly. I expected some of the same would happen from removing reshares, which I expected would make up over 20% of views under the status quo, and so would be filled in by more Groups content. For downranking sources with the same estimated ideology, I expected this would reduce exposure to political content, as much of the non-same-ideology posts will be by sources with estimated ideology in the middle of the range, i.e. [0.4, 0.6], which are less likely to be posting politics and hard news. I’ll also note that much of my uncertainty about how chronological ranking would perform was because there were a lot of unknown but important “details” about implementation, such as exactly how much of the ranking system really gets turned off (e.g., how much likely spam/scam content still gets filtered out in an early stage?).
How’d I do?
Here’s a quick summary of my guesses and the results in these three papers:
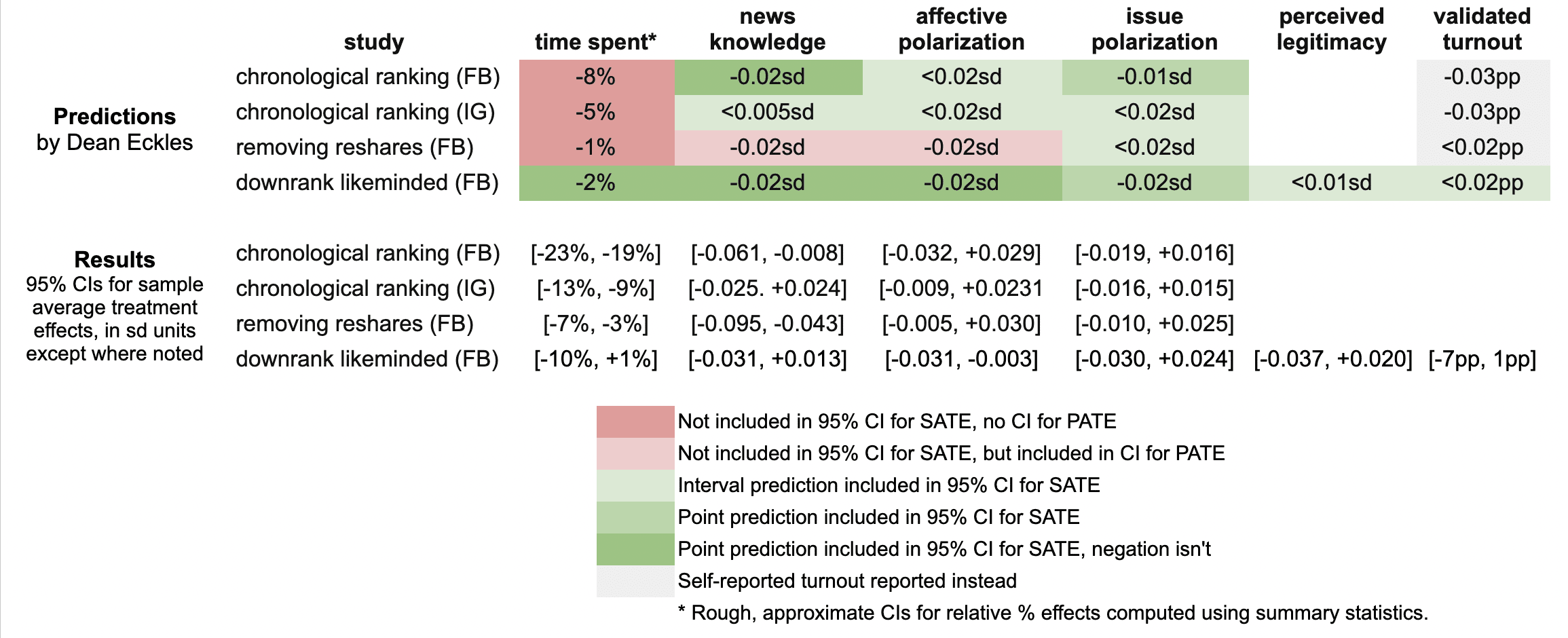
It looks like I was wrong in that the reductions in engagement were larger than I predicted: e.g., chronological ranking reduced time spent on Facebook by 21%, rather than the 8% I guessed, which was based on my background knowledge, a leaked report on a Facebook experiment, and this published experiment from Twitter.
Ex post I hypothesize that this is because of the duration of these experiments allowed for continual declines in use over months, with various feedback loops (e.g., users with chronological feed log in less, so they post less, so they get fewer likes and comments, so they log in even less and post even less). As I dig into the 100s of pages of supplementary materials, I’ll be looking to understand what these declines looked like at earlier points in the experiment, such as by election day.
My estimates for the survey-based outcomes of primary interest, such as polarization, were mainly covered by the 95% confidence intervals, with the exception of two outcomes from the “no reshares” intervention.
One thing is that all these papers report weighted estimates for a broader population of US users (population average treatment effects, PATEs), which are less precise than the unweighted (sample average treatment effect, SATE) results. Here I focus mainly on the unweighted results, as I did not know there was going to be any weighting and these are also the more narrow, and thus riskier, CIs for me. (There seems to have been some mismatch between the outcomes listed in the talk I saw and what’s in the papers, so I didn’t make predictions for some reported primary outcomes and some outcomes I made predictions for don’t seem to be reported, or I haven’t found them in the supplements yet.)
Now is a good time to note that I basically predicted what psychologists armed with Jacob Cohen’s rules of thumb might call extrapolate to “minuscule” effect sizes. All my predictions for survey-based outcomes were 0.02 standard deviations or smaller. (Recall Cohen’s rules of thumb say 0.1 is small, 0.5 medium, and 0.8 large.)
Nearly all the results for these outcomes in these two papers were indistinguishable from the null (p > 0.05), with standard errors for survey outcomes at 0.01 SDs or more. This is consistent with my ex ante expectations that the experiments would face severe power problems, at least for the kind of effects I would expect. Perhaps by revealed preference, a number of other experts had different priors.
A rare p < 0.05 result is that that chronological ranking reduced news knowledge by 0.035 SDs with 95% CI [-0.061, -0.008], which includes my guess of -0.02 SDs. Removing reshares may have reduced news knowledge even more than chronological ranking — and by more than I guessed.
Even with so many null results I was still sticking my neck out a bit compared with just guessing zero everywhere, since in some cases if I had put the opposite sign my estimate wouldn’t have been in the 95% CI. For example, downranking “like-minded” sources produced a CI of [-0.031, 0.013] SDs, which includes my guess of -0.02, but not its negation. On the other hand, I got some of these wrong, where I guessed removing reshares would reduce affective polarization, but a 0.02 SD effect is outside the resulting [-0.005, +0.030] interval.
It was actually quite a bit of work to compare my predictions to the results because I didn’t really know a lot of key details about exact analyses and reporting choices, which strikingly even differ a bit across these three papers. So I might yet find more places where I can, with a lot of reading and a bit of arithmetic, figure out where else I may have been wrong. (Feel free to point these out.)
Further reflections
I hope that this helps to contextualize the present results with expert consensus — or at least my idiosyncratic expectations. I’ll likely write a bit more about these new papers and further work released as part of this project.
It was probably an oversight for me not to make any predictions about the observational paper looking at polarization in exposure and consumption of news media. I felt like I had a better handle on thinking about simple treatment effects than these measures, but perhaps that was all the more reason to make predictions. Furthermore, given the limited precision of the experiments’ estimates, perhaps it would have been more informative (and riskier) to make point predictions about these precisely estimated observational quantities.
[I want to note that I was an employee or contractor of Facebook (now Meta) from 2010 through 2017. I have received funding for other research from Meta, Meta has sponsored a conference I organize, and I have coauthored with Meta employees as recently as earlier this month. I was also recently a consultant to Twitter, ending shortly after the Musk acquisition. You can find all my disclosures here.]
Does the “Table 1 fallacy” apply if it is Table S1 instead?
This post is cross-posted from Andrew Gelman’s Statistical Modeling, Causal Inference, and Social Science. There’s more discussion over there.
In a randomized experiment (i.e. RCT, A/B test, etc.) units are randomly assigned to treatments (i.e. conditions, variants, etc.). Let’s focus on Bernoulli randomized experiments for now, where each unit is independently assigned to treatment with probability q and to control otherwise.
Thomas Aquinas argued that God’s knowledge of the world upon creation of it is a kind of practical knowledge: knowing something is the case because you made it so. One might think that that in randomized experiments we have a kind of practical knowledge: we know that treatment was randomized because we randomized it. But unlike Aquinas’s God, we are not infallible, we often delegate, and often we are in the position of consuming reports on other people’s experiments.
So it is common to perform and report some tests of the null hypothesis that this process did indeed generate the data. For example, one can test that the sample sizes in treatment and control aren’t inconsistent with this. This is common in at least in the Internet industry (see, e.g., Kohavi, Tang & Xu on “sample ratio mismatch”), where it is often particularly easy to automate. Perhaps more widespread is testing whether the means of pre-treatment covariates in treatment and control are distinguishable; these are often called balance tests. One can do per-covariate tests, but if there are a lot of covariates then this can generate confusing false positives. So often one might use some test for all the covariates jointly at once.
Some experimentation systems in industry automate various of these tests and, if they reject at, say, p < 0.001, show prominent errors or even watermark results so that they are difficult to share with others without being warned. If we’re good Bayesians, we probably shouldn’t give up on our prior belief that treatment was indeed randomized just because some p-value is less than 0.05. But if we’ve got p < 1e-6, then — for all but the most dogmatic prior beliefs that randomization occurred as planned — we’re going to be doubtful that everything is alright and move to investigate.
In my own digital field and survey experiments, we indeed run these tests. Some of my papers report the results, but I know there’s at least one that doesn’t (though we did the tests) and another where we just state they were all not significant (and this can be verified with the replication materials). My sense is that reporting balance tests of covariate means is becoming even more of a norm in some areas, such as applied microeconomics and related areas. And I think that’s a good thing.
Interestingly, it seems that not everyone feels this way.
In particular, methodologists working in epidemiology, medicine, and public health sometimes refer to a “Table 1 fallacy” and advocate against performing and/or reporting these statistical tests. Sometimes the argument is specifically about clinical trials, but often it is more generally randomized experiments.
Stephen Senn argues in this influential 1994 paper:
Indeed the practice [of statistical testing for baseline balance] can accord neither with the logic of significance tests nor with that of hypothesis tests for the following are two incontrovertible facts about a randomized clinical trial:
1. over all randomizations the groups are balanced;
2. for a particular randomization they are unbalanced.
Now, no ‘significant imbalance’ can cause 1 to be untrue and no lack of a significant balance can make 2 untrue. Therefore the only reason to employ such a test must be to examine the process of randomization itself. Thus a significant result should lead to the decision that the treatment groups have not been randomized, and hence either that the trialist has practised deception and has dishonestly manipulated the allocation or that some incompetence, such as not accounting for all patients, has occurred.
In my opinion this is not the usual reason why such tests are carried out (I believe the reason is to make a statement about the observed allocation itself) and I suspect that the practice has originated through confused and false analogies with significance and hypothesis tests in general.
This highlights precisely where my view diverges: indeed the reason I think such tests should be performed is because I think that they could lead to the conclusion that “the treatment groups have not been randomized”. I wouldn’t say this always rises to the level of “incompetence” or “deception”, at least in the applications I’m familiar with. (Maybe I’ll write about some of these reasons at another time — some involve interference, some are analogous to differential attrition.)
It seems that experimenters and methodologists in social science and the Internet industry think that broken randomization is more likely, while methodologists mainly working on clinical trails put a very, very small prior probability on such events. Maybe this largely reflects the real probabilities in these areas, for various reasons. If so, part of the disagreement simply comes from cross-disciplinary diffusion of advice and overgeneralization. However, even some of the same researchers are sometimes involved in randomized experiments that aren’t subject to all the same processes as clinical trials.
Even if there is a small prior probability of broken randomization, if it is very easy to test for it, we still should. One nice feature of balance tests compared with other ways of auditing a randomization and data collection process is that they are pretty easy to take in as a reader.
But maybe there are other costs of conducting and reporting balance tests?
Indeed this gets at other reasons some methodologists oppose balance testing. For example, they argue that it fits into an, often vague, process of choosing estimators in a data-dependent way: researchers run the balance tests and make decisions about how to estimate treatment effects as a result.
This is articulated in a paper in The American Statistician by Mutz, Pemantle & Pham, which includes highlighting how discretion here creates a garden of forking paths. In my interpretation, the most considered and formalized arguments are saying is that conducting balance tests and then using that to determine which covariates to include in the subsequent analysis of treatment effects in randomized experiments has bad properties and shouldn’t be done. Here the idea is that when these tests provide some evidence against the null of randomization for some covariate, researchers sometimes then adjust for that covariate (when they wouldn’t have otherwise); and when everything looks balanced, researchers use this as a justification for using simple unadjusted estimators of treatment effects. I agree with this, and typically one should already specify adjusting for relevant pre-treatment covariates in the pre-analysis plan. Including them will increase precision.
I’ve also heard the idea that these balance tests in Table 1 confuse readers, who see a single p < 0.05 — often uncorrected for multiple tests — and get worried that the trial isn’t valid. More generally, we might think that Table 1 of a paper in a widely read medical journal isn’t the right place for such information. This seems right to me. There are important ingredients to good research that don’t need to be presented prominently in a paper, though it is important to provide information about them somewhere readily inspectable in the package for both pre- and post-publication peer review.
In light of all this, here is a proposal:
- Papers on randomized experiments should report tests of the null hypothesis that treatment was randomized as specified. These will often include balance tests, but of course there are others.
- These tests should follow the maxim “analyze as you randomize“, both accounting for any clustering or blocking/stratification in the randomization and any particularly important subsetting of the data (e.g., removing units without outcome data).
- Given a typically high prior belief that randomization occurred as planned, authors, reviewers, and readers should certainly not use p < 0.05 as a decision criterion here.
- If there is evidence against randomization, authors should investigate, and may often be able to fully or partially fix the problem long prior to peer review (e.g., by including improperly discarded data) or in the paper (e.g., by identifying the problem only affected some units’ assignments, bounding the possible bias).
- While it makes sense to mention them in the main text, there is typically little reason — if they don’t reject with a tiny p-value — for them to appear in Table 1 or some other prominent position in the main text, particularly of a short article. Rather, they should typically appear in a supplement or appendix — perhaps as Table S1 or Table A1.
This recognizes both the value of checking implications of one of the most important assumptions in randomized experiments and that most of the time this test shouldn’t cause us to update our beliefs about randomization much. I wonder if any of this remains controversial and why.
Marshaling the black ants: Practical knowledge in a small village in Malaysia
One of my favorite bits from James Scott’s Seeing Like a State:
While doing fieldwork in a small village in Malaysia, I was constantly struck by the breadth of my neighbors’ skills and their casual knowledge of local ecology. One particular anecdote is representative. Growing in the compound of the house in which I lived was a locally famous mango tree. Relatives and acquaintances would visit when the fruit was ripe in the hope of being given a few fruits and, more important, the chance to save and plant the seeds next to their own house. Shortly before my arrival, however, the tree had become infested with large red ants, which destroyed most of the fruit before it could ripen. It seemed nothing could be done short of bagging each fruit. Several times I noticed the elderly head of household, Mat Isa, bringing dried nipah palm fronds to the base of the mango tree and checking them. When I finally got around to asking what he was up to, he explained it to me, albeit reluctantly, as for him this was pretty humdrum stuff compared to our usual gossip. He knew that small black ants, which had a number of colonies at the rear of the compound, were the enemies of large red ants. He also knew that the thin, lancelike leaves of the nipah palm curled into long, tight tubes when they fell from the tree and died. (In fact, the local people used the tubes to roll their cigarettes.) Such tubes would also, he knew, be ideal places for the queens of the black ant colonies to lay their eggs. Over several weeks he placed dried nipah fronds in strategic places until he had masses of black-ant eggs beginning to hatch. He then placed the egg-infested fronds against the mango tree and observed the ensuing week-long Armageddon. Several neighbors, many of them skeptical, and their children followed the fortunes of the ant war closely. Although smaller by half or more, the black ants finally had the weight of numbers to prevail against the red ants and gain possession of the ground at the base of the mango tree. As the black ants were not interested in the mango leaves or fruits while the fruits were still on the tree, the crop was saved.
This successful field experiment in biological controls presupposes several kinds of knowledge: the habitat and diet of black ants, their egg-laying habits, a guess about what local material would substitute as movable egg chambers, and experience with the fighting proclivities of red and black ants. Mat Isa made it clear that such skill in practical entomology was quite widespread, at least among his older neighbors, and that people remembered something like this strategy having worked once or twice in the past. What is clear to me is that no agricultural extension official would have known the first thing about ants, let alone biological controls; most extension agents were raised in town and in any case were concerned entirely with rice, fertilizer, and loans. Nor would most of them think to ask; they were, after all, the experts, trained to instruct the peasant. It is hard to imagine this knowledge being created and maintained except in the context of lifelong observation and a relatively stable, multigenerational community that routinely exchanges and preserves knowledge of this kind. [Chapter 9]
Selecting effective means to any end
How are psychographic personalization and persuasion profiling different from more familiar forms of personalization and recommendation systems? A big difference is that they focus on selecting the “how” or the “means” of inducing you to an action — rather than selecting the “what” or the “ends”. Given the recent interest in this kind of personalization, I wanted to highlight some excerpts from something Maurits Kaptein and I wrote in 2010.1
This post excerpts our 2010 article, a version of which was published as:
Kaptein, M., & Eckles, D. (2010). Selecting effective means to any end: Futures and ethics of persuasion profiling. In International Conference on Persuasive Technology (pp. 82-93). Springer Lecture Notes in Computer Science.
For more on this topic, see these papers.
We distinguish between those adaptive persuasive technologies that adapt the particular ends they try to bring about and those that adapt their means to some end.
First, there are systems that use models of individual users to select particular ends that are instantiations of more general target behaviors. If the more general target behavior is book buying, then such a system may select which specific books to present.
Second, adaptive persuasive technologies that change their means adapt the persuasive strategy that is used — independent of the end goal. One could offer the same book and for some people show the message that the book is recommended by experts, while for others emphasizing that the book is almost out of stock. Both messages be may true, but the effect of each differs between users.
Example 2. Ends adaptation in recommender systems
Pandora is a popular music service that tries to engage music listeners and persuade them into spending more time on the site and, ultimately, subscribe. For both goals it is beneficial for Pandora if users enjoy the music that is presented to them by achieving a match between the music offering to individual, potentially latent music preferences. In doing so, Pandora adaptively selects the end — the actual song that is listened to and that could be purchased, rather than the means — the reasons presented for the selection of one specific song.
The distinction between end-adaptive persuasive technologies and means-adaptive persuasive technologies is important to discuss since adaptation in the latter case could be domain independent. In end adaptation, we can expect that little of the knowledge of the user that is gained by the system can be used in other domains (e.g. book preferences are likely minimally related to optimally specifying goals in a mobile exercise coach). Means adaptation is potentially quite the opposite. If an agent expects that a person is more responsive to authority claims than to other influence strategies in one domain, it may well be that authority claims are also more effective for that user than other strategies in a different domain. While we focus on novel means-adaptive systems, it is actually quite common for human influence agents adaptively select their means.
Influence Strategies and Implementations
Means-adaptive systems select different means by which to bring about some attitude or behavior change. The distinction between adapting means and ends is an abstract and heuristic one, so it will be helpful to describe one particular way to think about means in persuasive technologies. One way to individuate means of attitude and behavior change is to identify distinct influence strategies, each of which can have many implementations. Investigators studying persuasion and compliance-gaining have varied in how they individuate influence strategies: Cialdini [5] elaborates on six strategies at length, Fogg [8] describes 40 strategies under a more general definition of persuasion, and others have listed over 100 [16].
Despite this variation in their individuation, influence strategies are a useful level of analysis that helps to group and distinguish specific influence tactics. In the context of means adaptation, human and computer persuaders can select influence strategies they expect to be more effective that other influence strategies. In particular, the effectiveness of a strategy can vary with attitude and behavior change goals. Different influence strategies are most effective in different stages of the attitude to behavior continuum [1]. These range from use of heuristics in the attitude stage to use of conditioning when a behavioral change has been established and needs to be maintained [11]. Fogg [10] further illustrates this complexity and the importance of considering variation in target behaviors by presenting a two-dimensional matrix of 35 classes behavior change that vary by (1) the schedule of change (e.g., one time, on cue) and (2) the type of change (e.g., perform new behavior vs. familiar behavior). So even for persuasive technologies that do not adapt to individuals, selecting an influence strategy — the means — is important. We additionally contend that influence strategies are also a useful way to represent individual differences [9] — differences which may be large enough that strategies that are effective on average have negative effects for some people.
Example 4. Backfiring of influence strategies
John just subscribed to a digital workout coaching service. This system measures his activity using an accelerometer and provides John feedback through a Web site. This feedback is accompanied by recommendations from a general practitioner to modify his workout regime. John has all through his life been known as authority averse and dislikes the top-down recommendation style used. After three weeks using the service, John’s exercise levels have decreased.
Persuasion Profiles
When systems represent individual differences as variation in responses to influence strategies — and adapt to these differences, they are engaging in persuasion profiling. Persuasion profiles are thus collections of expected effects of different influence strategies for a specific individual. Hence, an individual’s persuasion profile indicates which influence strategies — one way of individuating means of attitude and behavior change — are expected to be most effective.
Persuasion profiles can be based on demographic, personality, and behavioral data. Relying primarily on behavioral data has recently become a realistic option for interactive technologies, since vast amounts of data about individuals’ behavior in response to attempts at persuasion are currently collected. These data describe how people have responded to presentations of certain products (e.g. e-commerce) or have complied to requests by persuasive technologies (e.g. the DirectLife Activity Monitor [12]).
Existing systems record responses to particular messages — implementations of one or more influence strategies — to aid profiling. For example, Rapleaf uses responses by a users’ friends to particular advertisements to select the message to present to that user [2]. If influence attempts are identified as being implementations of particular strategies, then such systems can “borrow strength” in predicting responses to other implementations of the same strategy or related strategies. Many of these scenarios also involve the collection of personally identifiable information, so persuasion profiles can be associated with individuals across different sessions and services.
Consequences of Means Adaptation
In the remainder of this paper we will focus on the implications of the usage of persuasion profiles in means-adaptive persuasive systems. There are two properties of these systems which make this discussion important:
1. End-independence: Contrary to profiles used by end-adaptive persuasive sys- tems the knowledge gained about people in means-adaptive systems can be used independent from the end goal. Hence, persuasion profiles can be used independent of context and can be exchanged between systems.
2. Undisclosed: While the adaptation in end-adaptive persuasive systems is often most effective when disclosed to the user, this is not necessarily the case in means-adaptive persuasive systems powered by persuasion profiles. Selecting a different influence strategy is likely less salient than changing a target behavior and thus will often not be noticed by users.
Although through the previous examples and the discussion of adaptive persuasive systems these two notions have already been hinted upon, we feel it is important to examine each in more detail.
End-Independence
Means-adaptive persuasive technologies are distinctive in their end-independence: a persuasion profile created in one context can be applied to bringing about other ends in that same context or to behavior or attitude change in a quite different context. This feature of persuasion profiling is best illustrated by contrast with end adaptation.
Any adaptation that selects the particular end (or goal) of a persuasive attempt is inherently context-specific. Though there may be associations between individual differences across context (e.g., between book preferences and political attitudes) these associations are themselves specific to pairs of contexts. On the other hand, persuasion profiles are designed and expected to be independent of particular ends and contexts. For example, we propose that a person’s tendency to comply more to appeals by experts than to those by friends is present both when looking at compliance to a medical regime as well as purchase decisions.
It is important to clarify exactly what is required for end-independence to obtain. If we say that a persuasion profile is end-independent than this does not imply that the effectiveness of influence strategies is constant across all contexts. Consistent with the results reviewed in section 3, we acknowledge that influence strategy effectiveness depends on, e.g., the type of behavior change. That is, we expect that the most effective influence strategy for a system to employ, even given the user’s persuasion profile, would depend on both context and target behavior. Instead, end-independence requires that the difference between the average effect of a strategy for the population and the effect of that strategy for a specific individual is relatively consistent across contexts and ends.
Implications of end-independence.
From end-independence, it follows that persuasion profiles could potentially be created by, and shared with, a number of systems that use and modify these profiles. For example, the profile constructed from observing a user’s online shopping behavior can be of use in increasing compliance in saving energy. Behavioral measures in latter two contexts can contribute to refining the existing profile.2
Not only could persuasion profiles be used across contexts within a single organization, but there is the option of exchanging the persuasion profiles between corporations, governments, other institutions, and individuals. A market for persuasion profiles could develop [9], as currently exists for other data about consumers. Even if a system that implements persuasion profiling does so ethically, once constructed the profiles can be used for ends not anticipated by its designers.
Persuasion profiles are another kind of information about individuals collected by corporations that individuals may or have effective access to. This raises issues of data ownership. Do individuals have access to their complete persuasion profiles or other indicators of the contents of the profiles? Are individuals compensated for this valuable information [14]? If an individual wants to use Amazon’s persuasion profile to jump-start a mobile exercise coach’s adaptation, there may or may not be technical and/or legal mechanisms to obtain and transfer this profile.
Non-disclosure
Means-adaptive persuasive systems are able and likely to not disclose that they are adapting to individuals. This can be contrasted with end adaptation, in which it is often advantageous for the agent to disclose the adaption and potentially easy to detect. For example, when Amazon recommends books for an individual it makes clear that these are personalized recommendations — thus benefiting from effects of apparent personalization and enabling presenting reasons why these books were recommended. In contrast, with means adaptation, not only may the results of the adaptation be less visible to users (e.g. emphasizing either “Pulitzer Prize winning” or “International bestseller”), but disclosure of the adaptation may reduce the target attitude or behavior change.
It is hypothesized that the effectiveness of social influence strategies is, at least partly, caused by automatic processes. According to dual-process models [4], un- der low elaboration message variables manipulated in the selection of influence strategies lead to compliance without much thought. These dual-process models distinguish between central (or systematic) processing, which is characterized by elaboration on and consideration of the merits of presented arguments, and pe- ripheral (or heuristic) processing, which is characterized by responses to cues as- sociated with, but peripheral to the central arguments of, the advocacy through the application of simple, cognitively “cheap”, but fallible rules [13]. Disclosure of means adaptation may increase elaboration on the implementations of the selected influence strategies, decreasing their effectiveness if they operate primarily via heuristic processing. More generally, disclosure of means adaptation is a disclosure of persuasive intent, which can increase elaboration and resistance to persuasion.
Implications of non-disclosure. The fact that persuasion profiles can be obtained and used without disclosing this to users is potentially a cause for concern. Potential reductions in effectiveness upon disclosure incentivize system designs to avoid disclosure of means adaptation.
Non-disclosure of means adaptation may have additional implications when combined with value being placed on the construction of an accurate persuasion profile. This requires some explanation. A simple system engaged in persuasion profiling could select influence strategies and implementations based on which is estimated to have the largest effect in the present case; the model would thus be engaged in passive learning. However, we anticipate that systems will take a more complex approach, employing active learning techniques [e.g., 6]. In active learning the actions selected by the system (e.g., the selection of the influence strategy and its implementation) are chosen not only based on the value of any resulting attitude or behavior change but including the value predicted improvements to the model resulting from observing the individual’s response. Increased precision, generality, or comprehensiveness of a persuasion profile may be valued (a) because the profile will be more effective in the present context or (b) because a more precise profile would be more effective in another context or more valuable in a market for persuasion profiles.
These later cases involve systems taking actions that are estimated to be non-optimal for their apparent goals. For example, a mobile exercise coach could present a message that is not estimated to be the most effective in increasing overall activity level in order to build a more precise, general, or comprehensive persuasion profile. Users of such a system might reasonably expect that it is designed to be effective in coaching them, but it is in fact also selecting actions for other reasons, e.g., selling precise, general, and comprehensive persuasion profiles is part of the company’s business plan. That is, if a system is designed to value constructing a persuasion profile, its behavior may differ substantially from its anticipated core behavior.
[1] Aarts, E.H.L., Markopoulos, P., Ruyter, B.E.R.: The persuasiveness of ambient intelligence. In: Petkovic, M., Jonker, W. (eds.) Security, Privacy and Trust in Modern Data Management. Springer, Heidelberg (2007)
[2] Baker, S.: Learning, and profiting, from online friendships. BusinessWeek 9(22) (May 2009)Selecting Effective Means to Any End 93
[3] Berdichevsky, D., Neunschwander, E.: Toward an ethics of persuasive technology. Commun. ACM 42(5), 51–58 (1999)
[4] Cacioppo, J.T., Petty, R.E., Kao, C.F., Rodriguez, R.: Central and peripheral routes to persuasion: An individual difference perspective. Journal of Personality and Social Psychology 51(5), 1032–1043 (1986)
[5] Cialdini, R.: Influence: Science and Practice. Allyn & Bacon, Boston (2001)
[6] Cohn,D.A., Ghahramani,Z.,Jordan,M.I.:Active learning with statistical models. Journal of Artificial Intelligence Research 4, 129–145 (1996)
[7] Eckles, D.: Redefining persuasion for a mobile world. In: Fogg, B.J., Eckles, D. (eds.) Mobile Persuasion: 20 Perspectives on the Future of Behavior Change. Stanford Captology Media, Stanford (2007)
[8] Fogg, B.J.: Persuasive Technology: Using Computers to Change What We Think and Do. Morgan Kaufmann, San Francisco (2002)
[9] Fogg, B.J.: Protecting consumers in the next tech-ade, U.S. Federal Trade Commission hearing (November 2006), http://www.ftc.gov/bcp/workshops/techade/pdfs/transcript_061107.pdf
[10] Fogg,B.J.:The behavior grid: 35 ways behavior can change. In: Proc. of Persuasive Technology 2009, p. 42. ACM, New York (2009)
[11] Kaptein, M., Aarts, E.H.L., Ruyter, B.E.R., Markopoulos, P.: Persuasion in am- bient intelligence. Journal of Ambient Intelligence and Humanized Computing 1, 43–56 (2009)
[12] Lacroix, J., Saini, P., Goris, A.: Understanding user cognitions to guide the tai- loring of persuasive technology-based physical activity interventions. In: Proc. of Persuasive Technology 2009, vol. 350, p. 9. ACM, New York (2009)
[13] Petty, R.E., Wegener, D.T.: The elaboration likelihood model: Current status and controversies. In: Chaiken, S., Trope, Y. (eds.) Dual-process theories in social psychology, pp. 41–72. Guilford Press, New York (1999)
[14] Prabhaker, P.R.: Who owns the online consumer? Journal of Consumer Market- ing 17, 158–171 (2000)
[15] Rawls, J.: The independence of moral theory. In: Proceedings and Addresses of the American Philosophical Association, vol. 48, pp. 5–22 (1974)
[16] Rhoads, K.: How many influence, persuasion, compliance tactics & strategies are there? (2007), http://www.workingpsychology.com/numbertactics.html
[17] Schafer, J.B., Konstan, J.A., Riedl, J.: E-commerce recommendation applications. Data Mining and Knowledge Discovery 5(1/2), 115–153 (2001)
- We were of course influenced by B.J. Fogg’s previous use of the term ‘persuasion profiling’, including in his comments to the Federal Trade Commission in 2006. [↩]
- This point can also be made in the language of interaction effects in analysis of variance: Persuasion profiles are estimates of person–strategy interaction effects. Thus, the end-independence of persuasion profiles requires not that the two-way strategy– context interaction effect is small, but that the three-way person–strategy–context interaction is small. [↩]
Is thinking about monetization a waste of our best minds?
I just recently watched this talk by Jack Conte, musician, video artist, and cofounder of Patreon:
Jack dives into how rapidly the Internet has disrupted the business of selling reproducible works, such as recorded music, investigative reporting, etc. And how important — and exciting — it is build new ways for the people who create these works to be able to make a living doing so. Of course, Jack has some particular ways of doing that in mind — such as subscriptions and subscription-like patronage of artists, such as via Patreon.
But this also made me think about this much-repeated1 quote from Jeff Hammerbacher (formerly of Facebook, Cloudera, and now doing bioinformatics research):
“The best minds of my generation are thinking about how to make people click ads. That sucks.”
I certainly agree that many other types of research can be very important and impactful, and often more so than working on data infrastructure, machine learning, market design, etc. for advertising. However, Jack Conte’s talk certainly helped make the case for me that monetization of “content” is something that has been disrupted already but needs some of the best minds to figure out new ways for creators of valuable works to make money.
Some of this might be coming up with new arrangements altogether. But it seems like this will continue to occur partially through advertising revenue. Jack highlights how little ad revenue he often saw — even as his videos were getting millions of views. And newspapers’ have been less able to monetize online attention through advertising than they had been able to in print.
Some of this may reflect that advertising dollars were just really poorly allocated before. But improving this situation will require a mix of work on advertising — certainly beyond just getting people to click on ads — such as providing credible measurement of the effects and ROI of advertising, improving targeting of advertising, and more.
Another side of this question is that advertising remains an important part of our culture and force for attitude and behavior change. Certainly looking back on 2016 right now, many people are interested in what effects political advertising had.
So maybe it isn’t so bad if at least some of our best minds are working on online advertising.
- So often repeated that Hammerbacher said to Charlie Rose, “That’s going to be on my tombstone, I think.” [↩]